In this talk we describe recent results in model building and selection
based ona formal definition of complexity and information in a given
data sequence, relative to a class of parametric models. These define a
universal parameter free model for the class, which factors into two
parts, one that has (asymptotically) all the useful information in the
data that can be extracted with the class of models and the
second defining noninformative noise. For the exponential classes the
factorization separates the noise perfectly for any amount of data,
defining a universal sufficient statistics decomposition in
generalization of the usual factorization of the likelihood function for
the exponential family. In particular, for the normal family in the
regression problemthis decomposition provides a powerful and natural
denoising algorithm, where noise is defined as the portion in the data
that cannot be compressed with the model class selected.
The universal model can be computed as the solution to a minmax problem,
whichis to find a code whose mean length is closest to the mean of the
ideal target, defined by the negative logarithm of the maximized
likelihood, where the mean is taken with respect to the worst case data
generating model lying outside of the parametric class. Moreover, for
the exponential class the minmax value is the norm, which can be
beaten only for a vanishing fraction of the best data generating models.
When Akaike's quest for the model in a parametric class, which is
closest in Kullback-Leibler distance to the data generating model outside
the class, is changed into finding the closest universal model, the
criterion becomes the MDL criterion rather than the inconsistent
AIC.
Abstract is PostScript format
Jorma Rissanen received his Ph.D. from the Technical University of
Helsinki in 1965. Since 1960 he has worked with IBM Research on
information theory, estimation and statistical inference and his work
in compression and universal modeling are widely cited, in particular
the work on Minimun Description Length (MDL) principle. He is a
recipient of
the IEEE Richard W. Hamming Award and was honored for receiving one of
the IEEE Information Theory Society's 1998 Golden Jubilee Awards for
Technological Innovation. The Golden Jubilee Awards are given to the
authors of discoveries, advances and inventions that have had a
profound impact in the technology of information transmission,
processing and compression. Dr. Rissanen was recognized for his
invention of arithmetic coding.
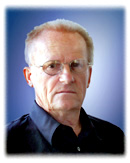
Jorma Rissanen